Contribution of low-cost sensor measurements to the prediction of PM2.5 levels: A case study in Imperial County, California, USA
Publication Date
1-1-2020
Document Type
Article
Publication Title
Environmental Research
Volume
180
DOI
10.1016/j.envres.2019.108810
Abstract
Regulatory monitoring networks are often too sparse to support community-scale PM2.5 exposure assessment while emerging low-cost sensors have the potential to fill in the gaps. To date, limited studies, if any, have been conducted to utilize low-cost sensor measurements to improve PM2.5 prediction with high spatiotemporal resolutions based on statistical models. Imperial County in California is an exemplary region with sparse Air Quality System (AQS) monitors and a community-operated low-cost network entitled Identifying Violations Affecting Neighborhoods (IVAN). This study aims to evaluate the contribution of IVAN measurements to the quality of PM2.5 prediction. We adopted the Random Forest algorithm to estimate daily PM2.5 concentrations at a 1-km spatial resolution using three different PM2.5 datasets (AQS-only, IVAN-only, and AQS/IVAN combined). The results show that the integration of low-cost sensor measurements is an effective way to significantly improve the quality of PM2.5 prediction with an increase of cross-validation (CV) R2 by ~0.2. The IVAN measurements also contributed to the increased importance of emission source-related covariates and more reasonable spatial patterns of PM2.5. The remaining uncertainty in the calibrated IVAN measurements could still cause apparent outliers in the prediction model, highlighting the need for more effective calibration or integration methods to relieve its negative impact.
Funding Number
R01ES022722
Funding Sponsor
National Institutes of Health
Keywords
Low-cost sensor, Measurement uncertainty, Random forest, Satellite AOD
Department
Meteorology and Climate Science
Recommended Citation
Jianzhao Bi, Jennifer Stowell, Edmund Y.W. Seto, Paul B. English, Mohammad Z. Al-Hamdan, Patrick L. Kinney, Frank R. Freedman, and Yang Liu. "Contribution of low-cost sensor measurements to the prediction of PM2.5 levels: A case study in Imperial County, California, USA" Environmental Research (2020). https://doi.org/10.1016/j.envres.2019.108810
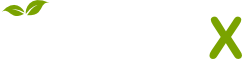
- Citations
- Citation Indexes: 64
- Policy Citations: 1
- Usage
- Abstract Views: 1
- Captures
- Readers: 105