Publication Date
4-1-2023
Document Type
Article
Publication Title
Computers and Security
Volume
127
DOI
10.1016/j.cose.2023.103098
Abstract
The anonymous nature of darknets is commonly exploited for illegal activities. Previous research has employed machine learning and deep learning techniques to automate the detection of darknet traffic in an attempt to block these criminal activities. This research aims to improve darknet traffic detection by assessing a wide variety of machine learning and deep learning techniques for the classification of such traffic and for classification of the underlying application types. We find that a Random Forest model outperforms other state-of-the-art machine learning techniques used in prior work with the CIC-Darknet2020 dataset. To evaluate the robustness of our Random Forest classifier, we obfuscate select application type classes to simulate realistic adversarial attack scenarios. We demonstrate that our best-performing classifier can be degraded by such attacks, and we consider ways to effectively deal with such adversarial attacks.
Keywords
Adversarial attacks, Auxiliary-Classifier generative adversarial network, Classification, Convolutional neural network, Darknet, Random forest
Creative Commons License
This work is licensed under a Creative Commons Attribution 4.0 License.
Department
Computer Science
Recommended Citation
Nhien Rust-Nguyen, Shruti Sharma, and Mark Stamp. "Darknet traffic classification and adversarial attacks using machine learning" Computers and Security (2023). https://doi.org/10.1016/j.cose.2023.103098
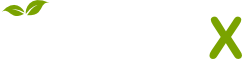
- Citations
- Citation Indexes: 38
- Usage
- Downloads: 167
- Abstract Views: 46
- Captures
- Readers: 52
- Mentions
- News Mentions: 1
Comments
This is the Version of Record and can also be read online here.