Multi-Relational Data Characterization by Tensors: Perturbation Analysis
Publication Date
1-1-2023
Document Type
Article
Publication Title
IEEE Transactions on Knowledge and Data Engineering
Volume
35
Issue
1
DOI
10.1109/TKDE.2021.3087671
First Page
756
Last Page
769
Abstract
Data perturbation is deemed a common problem in data processing. It is often inevitable to avoid noisy or misleading data which may arise from real-world collection or model imprecision. Besides, when data privacy is concerned, data perturbation is used as a prevalent data-protection approach, which alters individual data in a way such that the summary statistics still remain more or less the same. Since many data-mining problems can be formulated as tensor equations for characterizing multi-relational data, the main focus of this work is to perform a new perturbation analysis of tensor equations. From our recent study on tensor inversion, we propose a new mathematical framework to invert an arbitrary tensor but the existing iterative algorithms cannot always do so. In this work, we will establish the theoretical tensor-perturbation analysis to quantify the crucial query performance in terms of normalized error-norm with respect to perturbation degree and condition number. The condition number can be taken as a new measure to determine how the solution of a tensor equation varies as the entries are perturbed. Information-retrieval experiments for conducting the perturbation analysis of the solutions to tensor equations over both artificial and real data are undertaken and studied finally.
Keywords
data perturbation, multi-relational data, perturbation analysis, Tensor equations, tensor inverse
Department
Applied Data Science
Recommended Citation
Shih Yu Chang and Hsiao Chun Wu. "Multi-Relational Data Characterization by Tensors: Perturbation Analysis" IEEE Transactions on Knowledge and Data Engineering (2023): 756-769. https://doi.org/10.1109/TKDE.2021.3087671
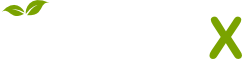
- Citations
- Citation Indexes: 8
- Usage
- Abstract Views: 86
- Captures
- Readers: 5
- Mentions
- News Mentions: 1