Publication Date
2-1-2023
Document Type
Article
Publication Title
Nuclear Engineering and Technology
Volume
55
Issue
2
DOI
10.1016/j.net.2022.10.032
First Page
603
Last Page
622
Abstract
Human error (HE) is an important concern in safety-critical systems such as nuclear power plants (NPPs). HE has played a role in many accidents and outage incidents in NPPs. Despite the increased automation in NPPs, HE remains unavoidable. Hence, the need for HE detection is as important as HE prevention efforts. In NPPs, HE is rather rare. Hence, anomaly detection, a widely used machine learning technique for detecting rare anomalous instances, can be repurposed to detect potential HE. In this study, we develop an unsupervised anomaly detection technique based on generative adversarial networks (GANs) to detect anomalies in manually collected surveillance data in NPPs. More specifically, our GAN is trained to detect mismatches between automatically recorded sensor data and manually collected surveillance data, and hence, identify anomalous instances that can be attributed to HE. We test our GAN on both a real-world dataset and an external dataset obtained from a testbed, and we benchmark our results against state-of-the-art unsupervised anomaly detection algorithms, including one-class support vector machine and isolation forest. Our results show that the proposed GAN provides improved anomaly detection performance. Our study is promising for the future development of artificial intelligence based HE detection systems.
Funding Number
DE-NE0008978
Funding Sponsor
U.S. Department of Energy
Keywords
anomaly detection, human error detection, machine learning, nuclear power plants
Creative Commons License
This work is licensed under a Creative Commons Attribution-Noncommercial-No Derivative Works 4.0 License.
Department
Marketing and Business Analytics
Recommended Citation
Ezgi Gursel, Bhavya Reddy, Anahita Khojandi, Mahboubeh Madadi, Jamie Baalis Coble, Vivek Agarwal, Vaibhav Yadav, and Ronald L. Boring. "Using artificial intelligence to detect human errors in nuclear power plants: A case in operation and maintenance" Nuclear Engineering and Technology (2023): 603-622. https://doi.org/10.1016/j.net.2022.10.032
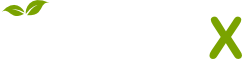
- Citations
- Citation Indexes: 25
- Usage
- Downloads: 158
- Abstract Views: 36
- Captures
- Readers: 78
- Mentions
- News Mentions: 2