Material removal rate prediction in chemical mechanical planarization with conditional probabilistic autoencoder and stacking ensemble learning
Publication Date
1-1-2022
Document Type
Article
Publication Title
Journal of Intelligent Manufacturing
DOI
10.1007/s10845-022-02040-w
Abstract
Chemical mechanical planarization (CMP) is a complex and high-accuracy polishing process that creates a smooth and planar material surface. One of the key challenges of CMP is to predict the material removal rate (MRR) accurately. With the development of artificial intelligence techniques, numerous data-driven models have been developed to predict the MRR in the CMP process. However, these methods are not capable of considering surface topography in MRR predictions because it is difficult to observe and measure the surface topography. To address this issue, we propose a graphical model and a conditional variational autoencoder to extract the features of surface topography in the CMP process. Moreover, process variables and the extracted features of surface topography are fed into an ensemble learning-based predictive model to predict the MRR. Experimental results have shown that the proposed method can predict the MRR accurately with a root mean squared error of 6.12 nm/min, and it outperforms physics-based machine learning and data-driven methods.
Keywords
Chemical mechanical planarization, Deep learning, Graphical model, Material removal rate prediction
Department
Industrial and Systems Engineering
Recommended Citation
Yupeng Wei and Dazhong Wu. "Material removal rate prediction in chemical mechanical planarization with conditional probabilistic autoencoder and stacking ensemble learning" Journal of Intelligent Manufacturing (2022). https://doi.org/10.1007/s10845-022-02040-w
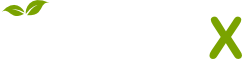
- Citations
- Citation Indexes: 11
- Usage
- Abstract Views: 16
- Captures
- Readers: 8
- Mentions
- News Mentions: 1