Publication Date
12-7-2020
Document Type
Article
Publication Title
ISPRS International Journal of Geo-Information
Volume
9
Issue
12
DOI
10.3390/ijgi9120732
Abstract
Crime prediction using machine learning and data fusion assimilation has become a hot topic. Most of the models rely on historical crime data and related environment variables. The activity of potential offenders affects the crime patterns, but the data with fine resolution have not been applied in the crime prediction. The goal of this study is to test the effect of the activity of potential offenders in the crime prediction by combining this data in the prediction models and assessing the prediction accuracies. This study uses the movement data of past offenders collected in routine police stop-and-question operations to infer the movement of future offenders. The offender movement data compensates historical crime data in a Spatio-Temporal Cokriging (ST-Cokriging) model for crime prediction. The models are implemented for weekly, biweekly, and quad-weekly prediction in the XT police district of ZG city, China. Results with the incorporation of the offender movement data are consistently better than those without it. The improvement is most pronounced for the weekly model, followed by the biweekly model, and the quad-weekly model. In sum, the addition of offender movement data enhances crime prediction, especially for short periods.
Keywords
crime prediction, historical crime, potential offenders, ST-Cokriging algorithm
Creative Commons License
This work is licensed under a Creative Commons Attribution 4.0 License.
Department
Urban and Regional Planning
Recommended Citation
Hongjie Yu, Lin Liu, Bo Yang, and Minxuan Lan. "Crime Prediction with Historical Crime and Movement Data of Potential Offenders Using a Spatio-Temporal Cokriging Method" ISPRS International Journal of Geo-Information (2020). https://doi.org/10.3390/ijgi9120732
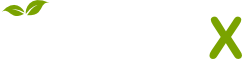
- Citations
- Citation Indexes: 16
- Usage
- Downloads: 88
- Abstract Views: 11
- Captures
- Readers: 41
- Mentions
- Blog Mentions: 1