Publication Date
4-1-2024
Document Type
Article
Publication Title
Sensors
Volume
24
Issue
7
DOI
10.3390/s24072295
Abstract
Anonymous networks, which aim primarily to protect user identities, have gained prominence as tools for enhancing network security and anonymity. Nonetheless, these networks have become a platform for adversarial affairs and sources of suspicious attack traffic. To defend against unpredictable adversaries on the Internet, detecting anonymous network traffic has emerged as a necessity. Many supervised approaches to identify anonymous traffic have harnessed machine learning strategies. However, many require access to engineered datasets and complex architectures to extract the desired information. Due to the resistance of anonymous network traffic to traffic analysis and the scarcity of publicly available datasets, those approaches may need to improve their training efficiency and achieve a higher performance when it comes to anonymous traffic detection. This study utilizes feature engineering techniques to extract pattern information and rank the feature importance of the static traces of anonymous traffic. To leverage these pattern attributes effectively, we developed a reinforcement learning framework that encompasses four key components: states, actions, rewards, and state transitions. A lightweight system is devised to classify anonymous and non-anonymous network traffic. Subsequently, two fine-tuned thresholds are proposed to substitute the traditional labels in a binary classification system. The system will identify anonymous network traffic without reliance on labeled data. The experimental results underscore that the system can identify anonymous traffic with an accuracy rate exceeding 80% (when based on pattern information).
Keywords
anonymous traffic, feature engineering, reinforcement learning, Tor, unsupervised learning
Creative Commons License
This work is licensed under a Creative Commons Attribution 4.0 License.
Department
Computer Engineering
Recommended Citation
Dazhou Liu and Younghee Park. "Anonymous Traffic Detection Based on Feature Engineering and Reinforcement Learning" Sensors (2024). https://doi.org/10.3390/s24072295
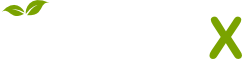
- Citations
- Citation Indexes: 1
- Usage
- Downloads: 8
- Abstract Views: 4
- Captures
- Readers: 3
- Mentions
- Blog Mentions: 1
- News Mentions: 1