Publication Date
9-1-2024
Document Type
Article
Publication Title
Information (Switzerland)
Volume
15
Issue
9
DOI
10.3390/info15090568
Abstract
Deep learning struggles with unsupervised tasks like community detection in networks. This work proposes the Enhanced Community Detection with Structural Information VGAE (VGAE-ECF) method, a method that enhances variational graph autoencoders (VGAEs) for community detection in large networks. It incorporates community structure information and edge weights alongside traditional network data. This combined input leads to improved latent representations for community identification via K-means clustering. We perform experiments and show that our method works better than previous approaches of community-aware VGAEs.
Keywords
community detection, deep learning, K-truss, Leiden algorithm, variational graph autoencoders
Creative Commons License
This work is licensed under a Creative Commons Attribution 4.0 License.
Department
Computer Science
Recommended Citation
Jyotika Hariom Patil, Petros Potikas, William B. Andreopoulos, and Katerina Potika. "Community Detection Using Deep Learning: Combining Variational Graph Autoencoders with Leiden and K-Truss Techniques" Information (Switzerland) (2024). https://doi.org/10.3390/info15090568
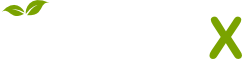
- Usage
- Downloads: 2
- Abstract Views: 1
- Captures
- Readers: 5
- Mentions
- Blog Mentions: 1
- News Mentions: 1