Publication Date
8-1-2024
Document Type
Article
Publication Title
Smart Cities
Volume
7
Issue
4
DOI
10.3390/smartcities7040088
First Page
2232
Last Page
2257
Abstract
Highlights: What are the main findings? The smart city community watch program developed using YOLOv5 for object detection and DeepSORT for multi-object tracking achieves 97% accuracy in detecting illegal dumping. The web-based application integrates person detection, trash detection, license plate detection and extraction, and a decision algorithm aiding government agencies to monitor and effectively manage illegal dumping. What are the implications of the main findings? With the 97% detection accuracy and real-time detection capabilities of the YOLOv5- and DeepSORT-based solution, this solution can help in saving government expenditure to clean up illegal dumping. The solution can be integrated with smart city programs such as smart waste management initiatives, aid in effective and proactive public management, and promote public health. The United States is the second-largest waste generator in the world, generating 4.9 pounds (2.2 kg) of Municipal Solid Waste (MSW) per person each day. The excessive amount of waste generated poses serious health and environmental risks, especially because of the prevalence of illegal dumping practices, including improper waste disposal in unauthorized areas. To clean up illegal dumping, the government spends approximately USD 600 per ton, which amounts to USD 178 billion per year. Municipalities face a critical challenge to detect and prevent illegal dumping activities. Current techniques to detect illegal dumping have limited accuracy in detection and do not support an integrated solution of detecting dumping, identifying the vehicle, and a decision algorithm notifying the municipalities in real-time. To tackle this issue, an innovative solution has been developed, utilizing a You Only Look Once (YOLO) detector YOLOv5 for detecting humans, vehicles, license plates, and trash. The solution incorporates DeepSORT for effective identification of illegal dumping by analyzing the distance between a human and the trash’s bounding box. It achieved an accuracy of 97% in dumping detection after training on real-time examples and the COCO dataset covering both daytime and nighttime scenarios. This combination of YOLOv5, DeepSORT, and the decision module demonstrates robust capabilities in detecting dumping. The objective of this web-based application is to minimize the adverse effects on the environment and public health. By leveraging advanced object detection and tracking techniques, along with a user-friendly web application, it aims to promote a cleaner, healthier environment for everyone by reducing improper waste disposal.
Funding Number
202304041101035
Keywords
big data, computer vision, deep learning, illegal dumping, scene classification, smart city, video surveillance
Creative Commons License
This work is licensed under a Creative Commons Attribution 4.0 License.
Department
Applied Data Science
Recommended Citation
Nupur Pathak, Gangotri Biswal, Megha Goushal, Vraj Mistry, Palak Shah, Fenglian Li, and Jerry Gao. "Smart City Community Watch—Camera-Based Community Watch for Traffic and Illegal Dumping" Smart Cities (2024): 2232-2257. https://doi.org/10.3390/smartcities7040088
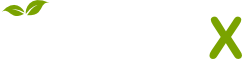
- Citations
- Citation Indexes: 1
- Usage
- Downloads: 6
- Abstract Views: 4
- Captures
- Readers: 32
- Mentions
- Blog Mentions: 1
- News Mentions: 1